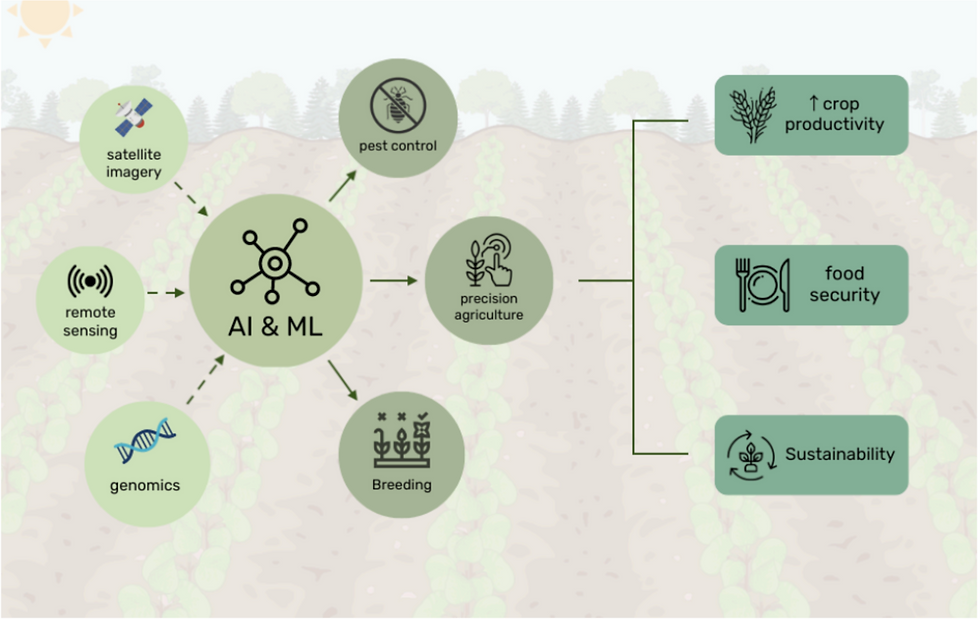
Graphical Abstract: different applications of Artificial Intelligence (AI) and Machine Learning (ML) in agriculture. Data from satellite imagery, remote sensing and genomics is used by AI and ML models to improve pest control, breeding and precision agriculture. As a result, crop productivity is increased, ultimately transforming agricultural practices and ensuring a more resilient and sustainable food production system.
Introduction
Nowadays, crop productivity is being negatively impacted by climate change and human activities through stressors such as droughts, pests and soil degradation. Moreover, the increasing world population poses a challenge for food security, placing extra burdens on agricultural resources (Pandey and Mishra 72). Therefore, tools like AI and machine learning offer potential solutions to enhance crop yield and overcome these challenges. Machine learning is a subcategory of AI that implements algorithms to recognize patterns and learn insights from data, enabling continuous improvement based on experience. Farmers can use AI to draw insights from their lands and, as a result, reduce fertilizers and pesticides and manage resources more efficiently. Additionally, advantageous agricultural traits, such as stress-resistance, may be discovered thanks to AI and used in crop breeding in a timely and cost-effective manner.
Breeding for improved crops
Adapting agricultural management to a changing climate is crucial for ensuring food security. AI can identify genetic traits that play a critical role in improving crop sustainability, yield, and nutrition, making crops resilient to extreme changes such as droughts and pests. Calice Biotech, for example, is an Argentine start-up that implements a combined approach using AI and bioinformatics to predict the results of various breedings and their performance in different environments. Thus, the company selects optimal plant genotypes for breeding, accelerating the development of improved crop varieties without GMOs (genetically modified organisms) and boosting crop yield while preventing economic losses for farmers (Agrofy News).
Precision agriculture
Data collected from various sources, such as sensors and satellite imagery, combined with AI can give farmers insights into their crops. For instance, by analyzing temperature variations in thermal images and other indicators, AI can predict areas likely to be affected by diseases or pest infestations before any symptoms appear (Van Loon). Hence, farmers can strategically apply treatments only in affected areas, avoiding the overuse of pesticides. John Deere is a company that uses space-based data and AI to pinpoint variations in nutrient levels and plant health, which allows farmers to make decisions in advance regarding fertilisation and crop protection, thereby increasing crop productivity (Mendonça).
Limitations of AI in agriculture
However, all of these AI implementations in agriculture are limited by digital literacy and inadequate infrastructure, especially in developing areas where farmers may lack the time and digital skills to implement AI on their own. Additionally, machine learning models and AI require a vast amount of data to be trained with, and although spatial data is easily obtained, crop-specific data is only available during specific times of the year, making the process of building robust machine learning models time-consuming (Javaid et al. 26).
Conclusion
Finally, despite being new in this field and the limitations it may encounter, the potential AI has to improve food security and reduce resource expenditure will revolutionize the agricultural field. Its ability to enhance crop yields, promote sustainable agricultural practices, and provide valuable insights to farmers will transform the agricultural landscape, ensuring a more resilient and efficient food production system for the future.
References
Agrofy News. “El grupo de biotecnológicos y científicos que asegura tener la fórmula para duplicar la producción de granos en Argentina”. Agrofy News. 2 Feb. 2024: https://news.agrofy.com.ar/noticia/208330/grupo-biotecnologicos-y-cientificos-que-asegur a-tener-formula-duplicar-produccion
Javaid, Mohd, et al. "Understanding the potential applications of Artificial Intelligence in Agriculture Sector", Advanced Agrochem, vol. 2, no. 1, (2023). pp. 15-30. Mendonça, Elaine. “John Deere Revolutionizes Agriculture with AI and Automation. Assembly. 6 June 2023:
https://www.assemblymag.com/articles/97831-john-deere-revolutionizes-agriculture-with ai-and-automation
Pandey, Dhananjay K., and Richa Mishra. "Towards sustainable agriculture: Harnessing AI for global food security." Artificial Intelligence in Agriculture, vol. 12, (2024). pp. 72-84 Van Loon, Ronald. “How AI is Driving Agricultural Innovation”. LinkedIn. 28 Nov. 2023: https://www.linkedin.com/pulse/how-ai-driving-agricultural-innovation-ronald-van-loon-j b27e
Article by: Pilar Leguiza
Comentários