Statistics can often be a scary subject for a lot of life scientists - it’s easy to let your eyes glaze over in confusion when reading over the stats section of a paper, or to zone out during a class whenever the topic comes up.
However, statistical analysis is a highly valuable tool in science; the increasing amounts of data that researchers have to handle in their work means that life science research and statistics often go hand in hand. An understanding of statistics enables researchers to design experiments that avoid bias, as well as successfully identify trends in the results and determine which differences between study groups are significant and which are simply cases of random variation.
This is really important in clinical trials when testing to see if a treatment is effective and can therefore be used to improve people’s lives; or in epidemiological studies that attempt to determine which groups are most at risk of developing a given disease, so the right advice can be given to those groups. Even when not involved directly in experimental design, everyone can benefit from an increased understanding of statistics when reading research papers, or even in everyday life!
For example, imagine you wake up one morning and look outside, but the sky is looking greyer than you hoped. You check the weather on your phone to look up the chance of rain and use that information to decide whether to hang the laundry out, or to bring an umbrella when you go outside.
Although you might only see a little percentage on your phone screen, behind that percentage there is a whole team of meteorologists working with complex statistical models to send out reports predicting what the skies will look like in a few days’ time. It is a great example of statistics in action.
While it’s easy to get overwhelmed by the sheer volume of advanced information and complex formulas when learning about statistics, this article will outline some of the most basic concepts those in life science should understand in the hope of making the topic a little less daunting and make it possible to embrace statistics for the game-changer it is.
Identifying what you’ve got: types of data
It’s important to understand what kind of data you’re working with to be able to analyse and present it correctly. It can be split into two main types.
Quantitative data
Quantitative data encompasses all things numerical, i.e. it expresses some kind of quantity or range. It can be categorised as continuous data (where the data can assume any value) or discrete data (where the data has distinct values).
For example, say ten students in a room all decide to use a tape measure to compare their heights. Since the students' actual heights can fall anywhere on the tape measure, this makes height an example of continuous data.
However, if one of the students gets mad and storms out of the room because they don’t want to admit that they’re the shortest (even though the numbers don’t lie), then there are nine people left. The number of students in the room counts as an example of discrete data, because the number of people in the room has to be a distinct value – you cannot have half a person leave the room (short jokes aside). Discrete data tends to be counted rather than measured.
If you're not quite sure what kind of quantitative data you’re dealing with, imagine a number line, with whole numbers marked out . Ask yourself – can my data have a value in between the marked out values? If the answer is yes, then your data is continuous, and if not, it is discrete.
Qualitative data
Qualitative data, on the other hand, is not quite so structured and covers data that describes characteristics that cannot really be quantified. For example, if you try to collect data about what type of cake everyone in your friend group likes best, you will not get values you can compare on a scale.
Qualitative data can also be split into two types: the cake example outlined above falls under the category of nominal data, where it is not possible to assign the values any particular order (and no, how much you personally like each of the cakes does not work as an order). Ordinal data is the other type – although this kind of data is still non-numerical, it is still possible to rank the data-for example, if you’re filling in a form and you’re asked about your level of education (where the categories might include: up to GCSEs, up to A-Levels and up to degree level).
Here is a handy chart to help you quickly distinguish between the different types of data you might come across:
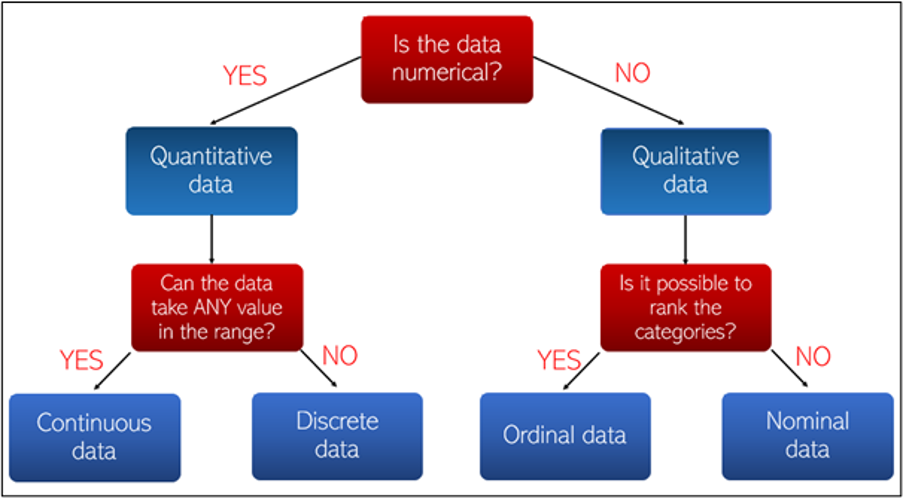
Figure 1: A flowchart showing how to differentiate between the different kinds of data. Figure adapted from https://www.graphpad.com/support/faq/what-is-the-difference-between-ordinal-interval-and-ratio-variables-why-should-i-care/
Summary statistics and data distribution
To learn more about a given data set at a glance, we often rely on descriptive or summary statistics, which provide useful information about the values in the data set.
Two examples of summary statistics which describe how large or small the data values are on average are the mean, which is defined as the average value of all the numbers in a data set, and the median, which is the middle value in a data set when all the values are ranked by size.
Descriptive statistics can also tell you how spread out the data values are in relation to each other. One example of this is standard deviation, which is the average distance of the individual values in the data set from from the overall mean value.
Another kind of statistic which is also used as a measure of dispersion is the interquartile range, which is the size of the difference between the largest and smallest value in the central 50% of the data. It is calculated by splitting a ranked data set into four equal groups, or “quartiles” based on the size of the individual values, and subtracting the value of the lower quartile (the value below which 25% of the values in the data set fall) from the upper quartile (the value above which 25% of the values in the data set fall). This method is further outlined here.
Which of the summary statistics are the most useful can depend on how the data set is distributed; a lot of biological data tends to follow a normal distribution (or Gaussian distribution), where most of the values fall in the middle of the range and values either side tend to be symmetrically distributed, meaning that mean and median are similar in value (as are standard deviation and interquartile range). As shown below, normal distributions on a graph follow the shape of a bell curve, in which about two-thirds of the data values fall within one standard deviation either side of the mean and approximately 95% of the data falls within two deviations.
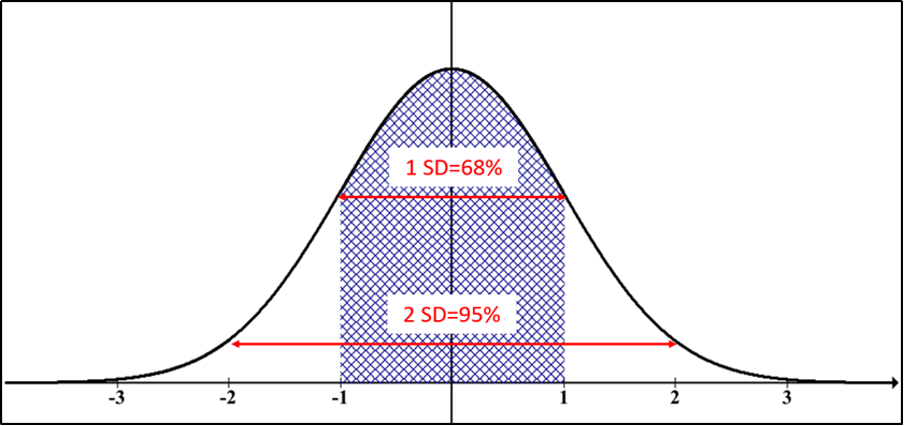
Figure 2: A normal distribution curve with standard deviations on the axis. The black line represents the mean and the red arrows represent the area of either 1 or two standard deviations (SD). Adapted from https://pixabay.com/vectors/distribution-normal-statistics-159626/
However, due to the sometimes unpredictable nature of biological systems, it is also possible for data sets to follow a totally different kind of distribution. In this case the mean and standard deviation may not be appropriate summary statistics to cite, since they can be easily skewed by extreme values (while median and interquartile range are less prone to this, because they are both found using the middle values of the data set rather than the largest or smallest values).
Figuring out whether data is normally distributed or not is also critical in applying the right kind of analysis, since a lot of statistical tests rely on the data assuming a normal distribution.
Inferential data, hypothesis testing and levels of significance
No matter what kind of statistical test you’re carrying out, the main aim is to answer the question: are any patterns that emerge from the data convincing enough to be trusted?
The answer isn’t always clear, since real life data tends to be somewhat chaotic. For example, although a clinical trial may try to demonstrate that a drug has a useful therapeutic effect, there are many other factors outside the control of the researchers that could also have an effect on a subject’s health (such as diet, exercise, other drugs they might be taking, etc.).
There are things the researchers can do to account for this, such as using a sufficiently large sample size. A general rule is that in a study large enough, the data moves towards a normal distribution and is therefore more likely to be representative of the general population. This is known as the central limit theorem.
However, this is not enough on its own, which is where rigorous hypothesis testing comes in.
Hypothesis testing relies on inferential statistics. Rather than simply describing the data, these statistics are used to draw a conclusion from a study that could be reliably applied to a wider population than what is focused on in the study itself.
Research experiments or studies aim to obtain results with enough statistical power to reject the null hypothesis: the proposal that in a given study, there is no difference between groups or that the observed variables do not influence one another in any way. An example of a null hypothesis might be: Intake of vitamin X has no impact on levels of protein Y in the blood.
Scientists often calculate (and constantly refer to) what is known as a P-value in their research. A P-value, or calculated probability, is the chance that the observed outcome has occurred due to chance (i.e. if the null hypothesis were true and the variables in the experiment had no impact on each other, but by coincidence, you just happened to obtain some results that look like they suggest otherwise at first glance).
It can also occur that, when calculated, the P-value comes to less than the set point of significance (the value commonly cited is 5%, or P=0.05), the data seems to be “statistically significant”, and it suggests that the null hypothesis is likely to be false, BUT…
Stats don’t tell the whole story!
Without context, statistics mean nothing.
There is nothing inherently special about a P-value of 0.05; this cut-off value is arbitrary and generally used out of convention. A P-value of less than 0.05 does not necessarily mean that the null hypothesis is proven to be false – it just provides evidence that this is the case. Conversely, a data set with a P-value of greater than 0.05 is not necessarily worthless – just because there is no effect that qualifies as “statistically significant” does not mean that there is absolutely no effect at all.
P-values are often paired with “confidence intervals”, which help to estimate the size of any effect that may have been shown by the data (since hypothesis testing alone does not tell you how large or small the effect is). A common value used is 95%, and in this context, 95% confidence limits mean that the mean has a 95% chance of falling between the calculated upper and lower confidence limits. But again, confidence limits are not infallible either.
If you have ever heard the phrase “correlation does not equal causation”, it absolutely applies here. Statistically, you might be able to show that there’s an association between two variables, but it doesn’t mean there is a causative relationship between them. A slightly strange example of this is shown in the graph below:
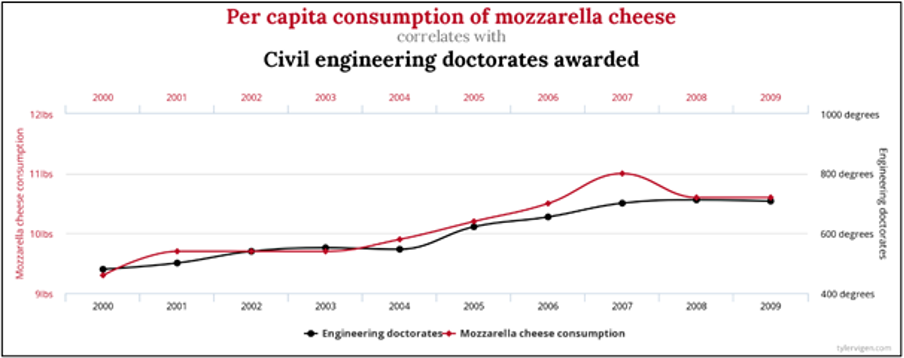
Figure 3: A caption showing per capita consumption of mozzarella cheese and how it correlates with the annual number of civil engineering doctorates awarded. Taken from https://www.tylervigen.com/spurious-correlations
Another thing to bear in mind is that statistics don’t indicate how valuable the study is overall; proper study design is still extremely important to avoid falling victim to fallacies such as the placebo effect, and an effect which is statistically significant may not necessarily be clinically useful. For example, even if a clinical trial shows that a new drug leads to a statistically significant improvement in disease symptoms relative to the placebo or an existing treatment, it may not be worth carrying out further study if the drug also causes an increase in harmful side effects.
No single experiment or study can tell you the truth about any observable scientific phenomena – all statements made off the back of statistics are probabilistic, and no statistical model will ever be able to tell the whole story. It is important to use the other information in the paper as well as the calculated statistics to decide for yourself if the data backs up the conclusion that the researchers behind a study have made.
Something important to note is that some researchers do occasionally manipulate statistics. This may not be as obvious as some of the clickbait articles you see on the internet, but sometimes data that doesn’t point to the desired conclusion is ignored or downplayed. Any papers which make claims that cannot be backed up with independently verified evidence should be taken with a pinch of salt.
The take-home message is that sometimes data can be difficult to sort through due to its sheer volume and complexity, and statistics can help us make a little more sense of it, but they aren’t infallible and thus shouldn’t just be blindly followed without a real understanding of what they mean. Instead, statistics should be treated as a valuable guide for navigating scientific phenomena and should be used in balance with common sense and good scientific judgement. It’s important to remember that the next step of a life science investigation that turns up promising results is to gather more evidence to support a theory through further study.
If you are interested in learning more about statistics in general, then these resources may be useful:
Plus, if you want to read about some more advanced statistics concepts applied to biology, then check out this collection of articles from Nature: Statistics For Biologists
Author: Emma McCarthy, MSc Health Data Science
コメント