The Role of Artificial Intelligence in the Future of Drug Discovery
- StemSide
- Nov 25, 2024
- 3 min read
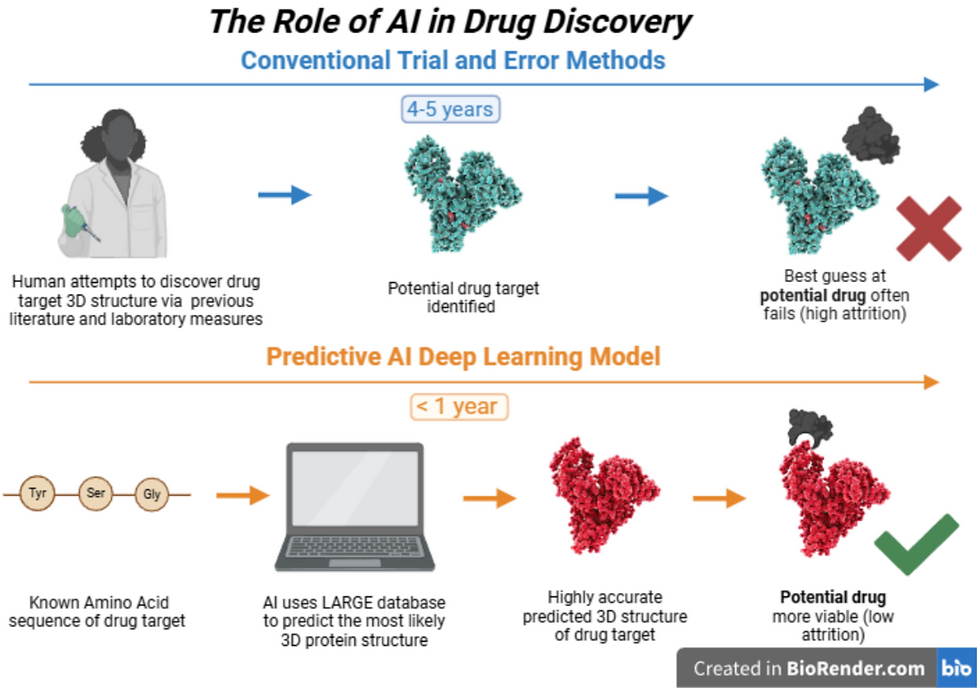
Graphical Abstract: Artificial Intelligence in the form of deep learning is being utilised to aid drug discovery through models such as AlphaFold. These methods can be quicker and cheaper overall than traditional drug discovery methods but still have some limitations.
The process of drug discovery is historically laborious, expensive and time-consuming with many drugs failing during drug development to show true efficacy. In recent years, there has been growing interest in using Artificial Intelligence, and specifically a subsection of machine learning called deep-learning, to tackle these issues and speed up the process of discovering lifesaving drugs. In this article I will discuss the current uses, benefits, challenges, and future of AI in our medicine.
A breakthrough in AI for biomedicine has been the development of the deep-learning model ‘AlphaFold’ which predicts unknown 3D structures of proteins from their amino acid sequence (Senior et al.) AlphaFold’s applications are broad but in terms of drug discovery, it could allow scientists to predict structures of potential drug targets and therefore better develop a complementary molecule that can ligate and cause desired effects in the body (Paul et al.) The importance of 3D protein structures in healthcare is illustrated by the COVID-19 pandemic, where researchers used shared databases cataloguing SARS-CoV-2 protein structures to help create effective treatments (Thornton et al.). While these treatments built on already available information from laboratories, it is highly likely future pandemics could involve completely unknown proteins, highlighting the importance of AlphaFold to quickly predict the structures of potential drug-protein binding sites.
As futuristic as it may seem, there are already many candidate drugs in development that were partially or fully discovered using AI. Namely, a new AI-created anticancer drug from biotech company Evotec is in phase 1 clinical trialling (Savage). Compared to usual discovery times of 4-5 years, the drug was discovered in an astonishing 8 months, highlighting the capability of AI to rapidly decrease the time taken to find effective drugs and then relay them to patients. Further, AlphaFold recently released thousands of potential psychedelics that could be used as antidepressants (Callaway).
Although undeniably promising, AI in drug discovery faces challenges along with scepticism from some researchers. One such challenge is the huge amounts of data required to initially train AI models (Blanco-González et al.). Once databases are sufficiently filled, the structure of a predicted protein could be up to 90% accurate (Lee et al.) However, when assessing molecules that are very under-studied, this accuracy can decrease. Indeed, there are several studies where AlphaFold predictions prove less accurate than protein structure visualisations through more established means like X-ray crystallography and cryo-electron microscopy (Terwilliger et al.)
Overall, AI can clearly aid drug discovery due to rapid roll-out speeds that allow drugs to meet patients sooner, preventing the many deaths that occur during the typically slow drug development process. With the unknown impacts of climate change on global disease, there is an ever heightened need to have AI measures that can safeguard our preparation against new pathogens. Nevertheless, it remains that AI cannot completely erase or replace current drug discovery methods and should instead be an extension to the toolkit of a pharmacologist. Thus, it is through utilising rather than over-relying on AI that we can effectively treat future disease.
References:
Blanco-González, Alexandre , et al. “The Role of AI in Drug Discovery: Challenges, Opportunities, and Strategies.” The Role of AI in Drug Discovery: Challenges, Opportunities, and Strategies, vol. 16, no. 6, 18 June 2023, pp. 891–891,
www.ncbi.nlm.nih.gov/pmc/articles/PMC10302890/, https://doi.org/10.3390/ph16060891.
Callaway, Ewen. “AlphaFold Found Thousands of Possible Psychedelics. Will Its Predictions Help Drug Discovery?” Nature, vol. 626, 18 Jan. 2024, www.nature.com/articles/d41586-024- 00130-8, https://doi.org/10.1038/d41586-024-00130-8.
Lee, Chien, et al. “Comparative Studies of AlphaFold, RoseTTAFold and Modeller: A Case Study Involving the Use of G-Protein-Coupled Receptors.” Briefings in Bioinformatics, vol. 23, no. 5, 9 Aug. 2022, https://doi.org/10.1093/bib/bbac308. Accessed 23 Oct. 2022.
Paul, Debleena, et al. “Artificial Intelligence in Drug Discovery and Development.” Drug Discovery Today, vol. 26, no. 1, Oct. 2020. ncbi,
Savage, Neil. “Tapping into the Drug Discovery Potential of AI.” Biopharma Dealmakers, 27 May 2021, www.nature.com/articles/d43747-021-00045-7, https://doi.org/10.1038/d43747-021- 00045-7.
Senior, Andrew W., et al. “Improved Protein Structure Prediction Using Potentials from Deep Learning.” Nature, vol. 577, no. 7792, Jan. 2020, pp. 706–710, https://doi.org/10.1038/s41586- 019-1923-7.
Terwilliger, Thomas C., et al. “AlphaFold Predictions Are Valuable Hypotheses and Accelerate but Do Not Replace Experimental Structure Determination.” Nature Methods, vol. 21, 30 Nov. 2023, pp. 1–7, www.nature.com/articles/s41592-023-02087-4, https://doi.org/10.1038/s41592- 023-02087-4.
Thornton, Janet M., et al. “AlphaFold Heralds a Data-Driven Revolution in Biology and Medicine.” Nature Medicine, vol. 27, no. 10, 1 Oct. 2021, pp. 1666–1669, www.nature.com/articles/s41591-021-01533-0, https://doi.org/10.1038/s41591-021-01533-0.
Article by: Tamsin Rowcliffe-Lucas